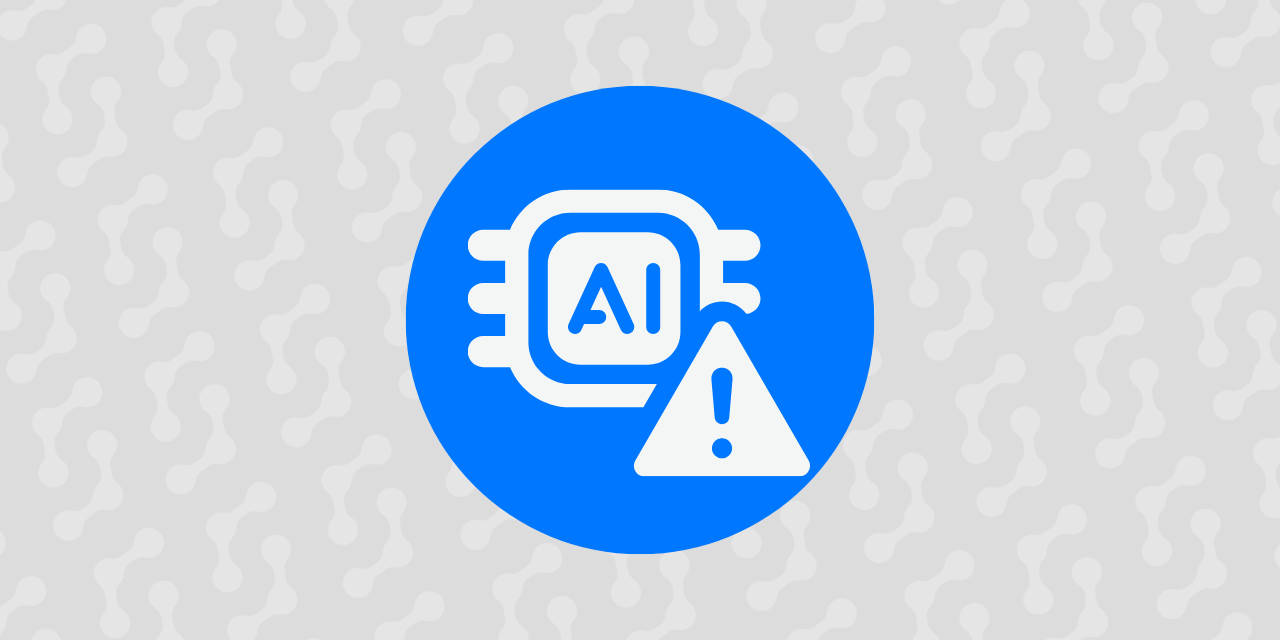
6 Common pitfalls when implementing AI Pricing Tools – and how to avoid them
04/09/2025 - Artificial Intelligence
Artificial Intelligence (AI) has emerged as a transformative force in retail, with huge potential to optimize pricing strategies. AI pricing tools promise retailers the ability to adjust prices dynamically, maximize profit margins, and respond quickly to market fluctuations. However, implementing these solutions does not come without its challenges. Gartner warns that 30% of AI projects will be abandoned after Proof of Concept (PoC). This underlines the importance of a solid strategy and an awareness of possible pitfalls before implementing an AI pricing tool.
This article explores the six most common mistakes companies make when adopting AI pricing tools, and, most importantly, provides practical strategies to help avoid them from day one. We will see how Reactev and its AI-powered Dynamic Pricing Software can be a vital tool in this process, helping retailers, vendors and direct-to-consumer brands overcome these challenges and unlock the full potential of AI in their pricing strategy.
1) Underestimating the importance of quality data
Why “garbage in, garbage out” also applies to pricing
AI is, in essence, powered by data. The ability to generate accurate insights and effective recommendations depends directly on the quality of the information received. If the data entered into the system is incomplete, inaccurate, inconsistent, or irrelevant, the results obtained will inevitably be poor. The “garbage in, garbage out” (GIGO) principle is fundamental to the context of AI pricing.
Imagine trying to predict demand for a product based on sales data that contains errors, or does not reflect market reality. However sophisticated AI is, it can’t compensate for these shortcomings, so the resulting pricing decisions could be counterproductive. Having a high-quality data provider, such as Minderest — specialized in monitoring pricing data, catalogues and stock in the retail sector — is key to successfully implementing AI pricing tools.
How to prepare your database for efficient AI
It is crucial to implement a robust data management strategy to ensure the success of your AI pricing project. This involves:
- Centralizing data: Gather all relevant data into a single repository, removing silos and facilitating access and analysis.
- Cleaning up inconsistencies: Identify and correct errors, outliers and missing data. Standardize formats and units of measurement.
- Feeding with external sources: Complement your internal data with market, competitor and economic trend data, as well as other relevant factors.
- Segmenting for accurate analysis: Divide your data into significant segments (by product, customer, location, etc.) for more detailed and personalized insights.
If you want to delve deeper into the relationship between data and pricing strategy, we recommend reading how to set a data-driven pricing strategy.
2) Implementing without clearly defined business objectives
Which metrics should guide your pricing project with AI?
Before implementing an AI pricing tool, you must clearly define your business objectives. Do you want to maximize profit margins, increase sales volume, gain market share or improve the elasticity of demand? Your key performance indicators (KPIs) must directly align with these objectives.
Some common KPIs in the context of AI pricing include:
- Profit margin: The difference between the selling price and the cost of the products.
- Sales volume: The quantity of products sold in a given period.
- Market share: The percentage of total market sales achieved by your company.
- Elasticity of demand: How demand is affected by price changes.
How to align technical objectives with financial results
Once you have defined your KPIs, it is crucial to translate business objectives into specific AI objectives. For example, if the goal is profit margin optimization, AI’s objective could be to identify the optimal prices that will allow the business to reach that margin, without adversely affecting sales volume.
Establishing a monitoring system to assess AI’s impact on financial results is vital. This involves regularly tracking KPIs and adjusting AI models as needed.
3) Thinking AI is a 100% autonomous solution
The fundamental role played by a human team
Although AI can automate many price analysis and adjustment tasks, it’s not a 100% stand-alone solution. The human team remains essential to interpret results, make strategic decisions, and adjust AI models as needed.
The pricing team’s experience and knowledge provide invaluable contributions by interpreting AI-generated insights. Their ability to understand market context, competitive dynamics and customer needs is critical to making sound pricing decisions.
Supervision, learning and continuous adjustments
Artificial Intelligence requires continuous monitoring and learning to ensure its effectiveness. Human feedback is essential to training AI, correcting errors, and adapting it to market and customer behaviour changes.
Establishing a regular review process for AI results and adjustments made by the pricing team is crucial. This will allow the team to identify areas for improvement and optimize the tool’s performance.
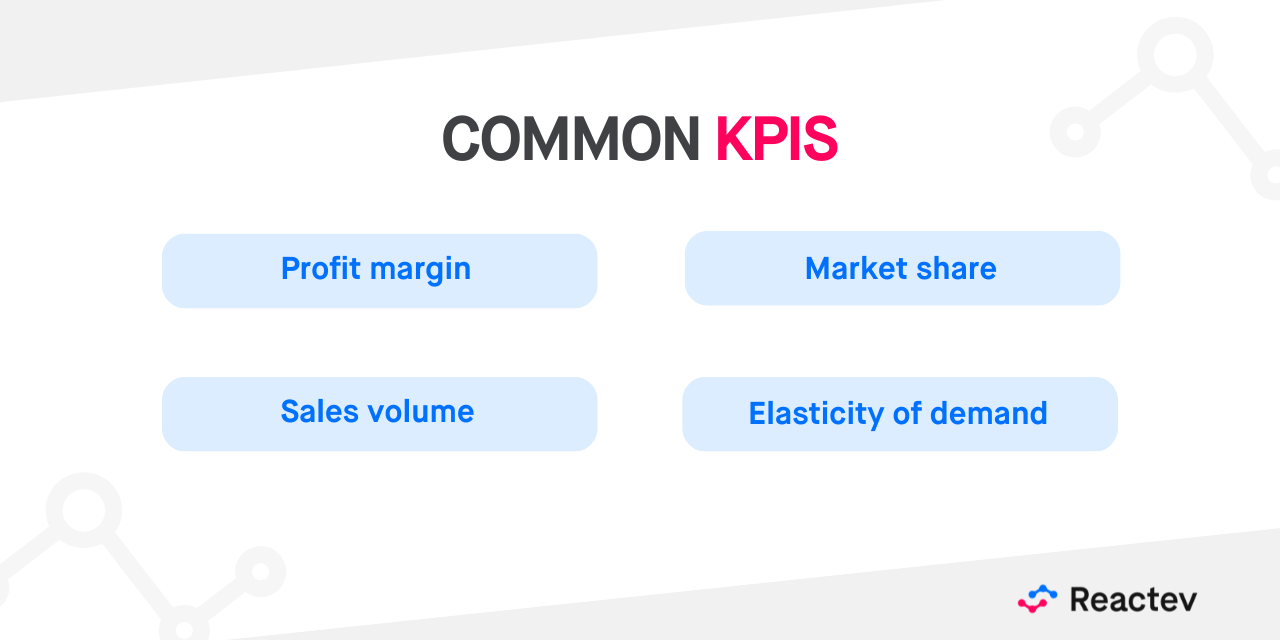
4) Ignoring the impact of change on pricing teams
How to manage internal resistance to AI use
Implementing an AI pricing tool can cause internal conflicts among pricing teams. Fear of automation and uncertainty about the future of their roles can prove to be major obstacles.
To manage this reticence, involve the team from day one of the project, clearly communicate the benefits of AI and provide training and support during the transition process.
Training and communication as key levers
Training is essential so that the pricing team can effectively use the new AI tool. It’s important to explain how AI works, what tasks it automates, and how it frees up time for higher-strategic-value activities, such as data analytics and pricing strategy planning.
Open and transparent communication is critical to building trust and reducing anxiety. Keeping the team updated about the project’s progress, results, and future plans is also essential.
5) Failing to adapt the model to your sector or type of product
Algorithms don’t all work the same way in retail: Fashion, food…
Each sector and type of product has different price dynamics and sensitivities. An AI model that works well for an electronics retailer may not be suitable for a fashion store or supermarket.
It is crucial to choose an AI pricing tool that can be adapted to your sector and product type’s specific needs. This involves considering factors such as seasonality, trends, elasticity of demand, competition and costs, to name but a few.
Examples of vertical personalization:
- Fashion: In the fashion sector, it is crucial to consider trends, seasonality, product lifecycles, and customer sensitivity to promotions.
- Food: In the food sector, product perishability, daily demand, promotions and local competitors, are key factors to consider.
- Electronics: Rapid technological developments, competitive pricing, and customer sensitivity to product characteristics and specifications are key factors in the electronics sector.
6) Evaluating the ROI too soon (or with the wrong KPIs)
When and how to measure the real impact of an AI tool
It is essential to be patient and establish a realistic timeline to assess an AI pricing tool’s return on investment (ROI). Results can take time to fully manifest, as AI needs time to learn and optimize its performance.
Using the right KPIs to measure AI’s impact is also crucial. You can’t just focus on a single indicator. You need to consider a series of metrics that reflect the tool’s overall impact on the business.
Recommended KPIs during implementation:
- Initial stage: During the early implementation stage, it is important to focus on data quality and the accuracy of AI models. Relevant KPIs in this phase may include the data error rate, the accuracy of AI forecasts, and the rate of adoption of the tool by the pricing team.
- Intermediate stage: As AI is optimized, measuring its impact on pricing decisions is important. Relevant KPIs at this stage may include the frequency of price adjustments, how prices align with business objectives, and how satisfied the pricing team are with the tool.
- Advanced stage: In the advanced implementation stage, it is vital to assess the impact of AI on the business’s financial results. Relevant KPIs at this stage may include profit margin, sales volume, market share and elasticity of demand.
How to guarantee success for your AI Pricing project
Successful implementation of AI in pricing strategies relies on thorough preparation, clearly defined objectives, collaboration between humans and machines, adaptation to specific business needs and continuous impact measurement.
With Reactev and its AI-powered Dynamic Pricing Software, retailers, sellers, and direct-to-consumer brands can overcome these challenges and unlock the full potential of AI to optimize their pricing strategies, increase profitability, and improve competitiveness.
Ready to implement AI in your pricing strategy? Request a Reactev demo and discover how we can help you transform your pricing.
Category: Artificial Intelligence